STS perspectives on the unfolding data revolution
Society finds itself at the beginning of a digital era where every device is online and sensors create continuous streams of data. The increased volume, velocity, and variety of this data is encompassed in the concept “big data”. The rise of big data has gone hand in hand with an ongoing increase in computational power which allows for the development of ever more sophisticated data analysis techniques, models, and algorithms. This broad collection of data-centric method innovations is referred to as “data science” (Hey, 2006). Although the concepts of big data and data science are loosely defined and sometimes used interchangeably, in this essay I adopt the distinction as outlined above.
Data science has quickly proliferated outside academia and has attracted interest – and substantial investment – in the public and private sector. Data science is applied in a diversity of substantive areas, including smart cities, smart maintenance, e-health, and e-commerce. Over the years, quantifications in a general sense have earned a reputation in some fields for outperforming human decision makers (Dawes, 1979). Achievements of data science, such as the victory of AlphaGo – a deep learning algorithm- over professional go player Lee Sedol, have attracted widespread media attention.
While much effort is devoted toward advancing technical data science capability, our understanding of the non-technical side to data science has lagged behind. Here, I use technical to broadly discern the quantitative and the non-quantitative elements of data science. This hiatus has caught the attention of several STS scholars; 4S/EASST featured tracks such as “The Potential Futures of Data Science: A Roundtable Intervention” and “Critical data studies”, amongst others. This demonstrates the growing interest from the STS community in data science. In this essay, I reflect on my visit to 4S/EASST Barcelona and by summarizing my fieldnotes and providing a short form digital ethnography.
Through the process of rearranging my 4S/EASST notes – and hastily captured photos of slides – different themes emerged. As a recent sociology PhD graduate, I found that data science brings into focus new challenges (e.g. data-ownership, transparency of artificial neural networks) as well as existing ones (e.g. biases inherent to quantifications). It also draws our attention towards some practical issues for conducting research (e.g. how to study a deep-learning algorithm?).
It is well beyond the scope of this text to discuss all, if any, these topics in detail. Instead, I focus on a challenge that is also relevant to practitioners: How are data scientists coming to terms with their vaguely delineated, yet increasingly topical field? In this context, what does it mean to be a data scientist? Being a practitioner myself, how do I know if am I genuinely a data scientist?
These questions are of interest to STS precisely because data science is an emerging field. To illustrate this point, I draw on material that I have come across in my work as a data scientist. I will discuss the differences and similarities between ‘genres’ that express some definition of data science or data scientists. Perhaps the most salient example of this is the multitude of Venn diagrams that are disseminated online. These diagrams aim to describe what skills or areas of expertise are covered by data science and which ones are not. Figure 1 juxtaposes two such Venn diagrams. Although there are overlaps between the two (e.g. ‘subject matter expertise’ and ‘domain expertise’), the
re are also differences. For example, the diagram on the left does not include the sphere of ‘Social Sciences’. The diagram on the right also marks some areas as ‘danger zones’. These zones are not just considered outside of data science as a field, but also seem to present these zones as combinations of skills that can be risky. The diagram on the left takes a different approach and gives the honorary title of ‘unicorn’ to the data scientist possessing all required skills.
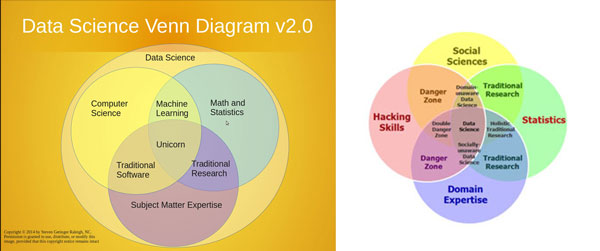
The material on definitions of data science is not limited to Venn diagrams. Another genre that can be identified is that of infographics, see Figure 2. These images differ from the Venn charts in that they do not represent the overlap between different areas. Nor do the explicitly state what combinations of skills can be considered dangerous. Rather, these combinations of text and art offer a list of skills that data scientists are expected to have or attain. Some of the skills listed were also present in the Venn diagrams. For example, ‘math and statistics’ can be seen in all of the images and ‘programming’ or ‘hacking’ in three out of four. The infographics seem to put more emphasis on ‘soft skills’ such as communication and project management.
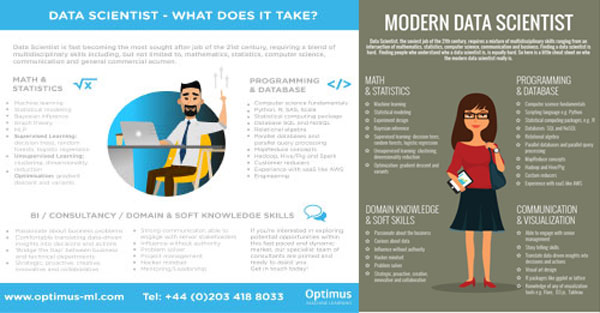
Online vacancies for data scientists are a third genre that deals with the definition of data science and data scientists. As with the previous two genres there are substantial differences between the two examples shown in Figure 3. The required skills in the left advert include programming languages and experience in bash. These skills are absent from the second advert. Instead, it asks for experience in spreadsheet software and work experience at one of the big consultants. There are similarities between the two, both adverts ask for skills in working with databases and experience with a – albeit different – set of technologies. Yet, the successful applicant to either vacancy can update his or her job title to “data scientist”.
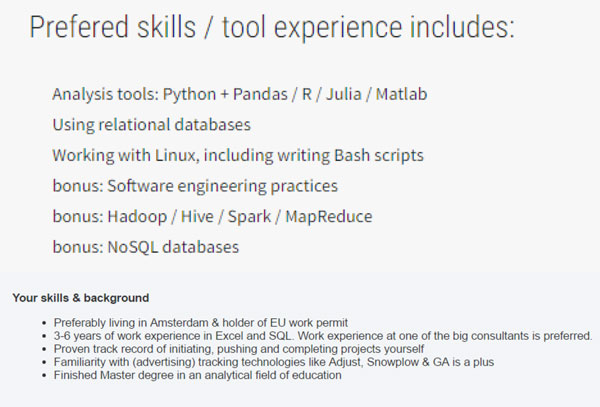
The three genres outlined above offer different styles that data scientists use to come to terms with their emerging field. The genres offer different styles of definitions of data science and delineate the profession of data scientist in different ways. Although cross-cutting skills can be identified, it would seem there is a wide diversity in what is currently understood as data science and consequentially there is little consensus on what it means to be a data scientist. To practitioners, it remains unclear on what grounds one can use the job title of ‘data scientist’ as the required skillset and experience is divergent. As a data science professional, I am cautious of using the term data scientist. When I introduce myself to a peer, I try to first establish a working consensus of the term by explaining what I do. It perhaps not surprising that new classifications are starting to emerge under the umbrella of ‘data professions’. For example, some are now discerning between, data engineers, data analysts, data solution consultants and data regulatory officers, to name a few.
This essay outlined several challenges and questions which emerged from the material presented at the 4S/EASST conference. I proceeded by illustrating one of these challenges – the definition of data science – by presenting some online material. The essay demonstrates that there exists no consensus amongst practitioners of data science regarding the boundaries of their field or the skillset that associated with ‘data scientists’. This is just one of the non-technical aspects of data science. With the abundance of funding that is allocated towards data science initiatives, it seems both opportune and important that we move to develop directions for research on data science in STS. Surely, data science will prove an interesting subject for STS scholars for years to come.